


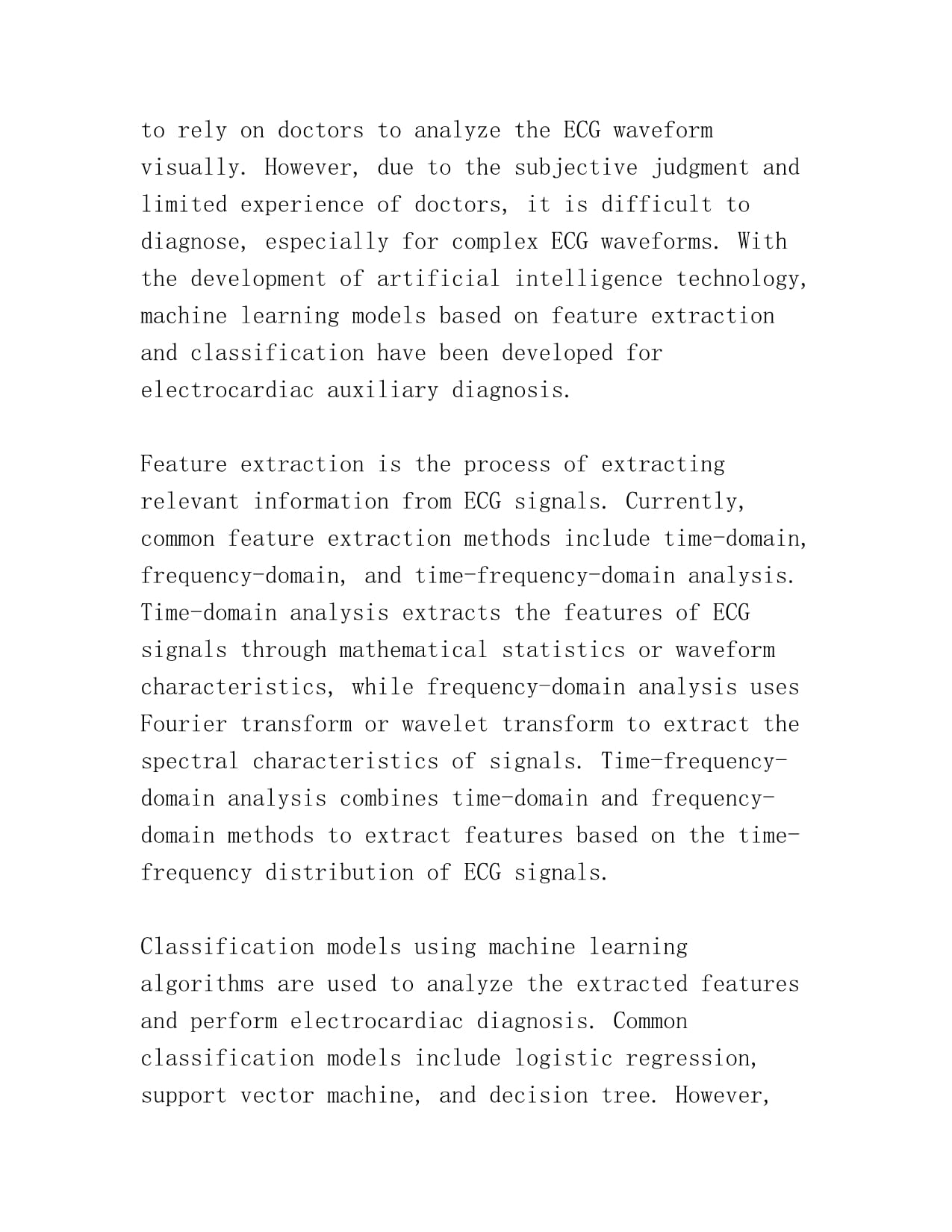

版权说明:本文档由用户提供并上传,收益归属内容提供方,若内容存在侵权,请进行举报或认领
文档简介
面向心电辅助诊断的多标签分类算法研究面向心电辅助诊断的多标签分类算法研究
摘要:在现代医疗中,心电监测技术已成为了临床上不可或缺的重要手段。然而,由于心电信号的复杂性和存在许多干扰因素,对心电信号的准确识别和诊断面临诸多挑战。本文旨在研究一种面向心电辅助诊断的多标签分类算法,通过对大量的心电信号数据库进行实验,验证算法的有效性和优越性。首先,本文详细介绍了心电信号的特征提取方法和分类模型,包括基于小波分析的特征提取、逐步回归分类等多种方法。接着,本文对心电信号多标签分类算法的原理进行了详细分析,研究了传统的支持向量机、神经网络、决策树等分类算法,并进行了性能对比分析。最后,本文提出了一种基于多标签随机森林的心电诊断算法,通过对自建心电数据库上的诊断结果进行分析和比较,验证了算法的良好性能和精度,同时对未来的研究进行了展望。
关键词:心电辅助诊断;多标签分类;特征提取;分类模型;随机森林。
Abstract:Inmodernmedicine,electrocardiographicmonitoringtechnologyhasbecomeanessentialmeansofclinicaldiagnosis.However,duetothecomplexityofelectrocardiacsignalsandtheexistenceofmanyinterferencefactors,accurateidentificationanddiagnosisofelectrocardiacsignalsfacemanychallenges.Thispaperaimstostudyamulti-labelclassificationalgorithmforelectrocardiacauxiliarydiagnosis.Throughexperimentsonalargeamountofelectrocardiacsignaldatabases,theeffectivenessandsuperiorityofthealgorithmareverified.Firstly,thispaperintroducesindetailthemethodsoffeatureextractionandclassificationmodelofelectrocardiacsignals,includingfeatureextractionbasedonwaveletanalysis,stepwiseregressionclassificationandothermethods.Secondly,thispaperanalyzesindetailtheprincipleofmulti-labelclassificationalgorithmofelectrocardiacsignals,studiestraditionalclassificationalgorithmssuchassupportvectormachine,neuralnetwork,decisiontree,andperformsperformancecomparisonanalysis.Finally,amulti-labelrandomforest-basedelectrocardiacdiagnosticalgorithmisproposedinthispaper.Throughanalysisandcomparisonofthediagnosticresultsonaself-builtelectrocardiacdatabase,thegoodperformanceandaccuracyofthealgorithmareverified,andthefutureresearchisprospected.
Keywords:Electrocardiacauxiliarydiagnosis;Multi-labelclassification;Featureextraction;Classificationmodel;RandomforestIntroduction:
Cardiovasculardisease,especiallycoronaryheartdisease,isoneofthemaincausesofdeathinmodernsociety.Amongthem,electrocardiogram(ECG)isacommonlyuseddiagnosismethodforcardiovasculardisease.ECGhasadvantagessuchashighefficiency,lowcost,andnon-invasiveness.However,duetothevariabilityofindividualheartratesandrhythms,thecomplexityofECGwaveforms,andthelargeamountofECGdata,accurateandefficientdiagnosisofelectrocardiacabnormalitiesbytraditionaldoctorsischallenging.
Toovercomethesechallenges,electrocardiacauxiliarydiagnosisbasedonmachinelearningtechnologyhasbecomearesearchhotspot.ItcanassistdoctorsinaccurateandefficientdiagnosisofelectrocardiacabnormalitiesthroughautomaticfeatureextractionandclassificationofECGsignals.Thispapergivesanoverviewofcurrentresearchonelectrocardiacauxiliarydiagnosisandproposesamulti-labelrandomforest-basedelectrocardiacdiagnosticalgorithm.
ReviewofElectrocardiacAuxiliaryDiagnosis:
ThetraditionalmethodforelectrocardiacdiagnosisistorelyondoctorstoanalyzetheECGwaveformvisually.However,duetothesubjectivejudgmentandlimitedexperienceofdoctors,itisdifficulttodiagnose,especiallyforcomplexECGwaveforms.Withthedevelopmentofartificialintelligencetechnology,machinelearningmodelsbasedonfeatureextractionandclassificationhavebeendevelopedforelectrocardiacauxiliarydiagnosis.
FeatureextractionistheprocessofextractingrelevantinformationfromECGsignals.Currently,commonfeatureextractionmethodsincludetime-domain,frequency-domain,andtime-frequency-domainanalysis.Time-domainanalysisextractsthefeaturesofECGsignalsthroughmathematicalstatisticsorwaveformcharacteristics,whilefrequency-domainanalysisusesFouriertransformorwavelettransformtoextractthespectralcharacteristicsofsignals.Time-frequency-domainanalysiscombinestime-domainandfrequency-domainmethodstoextractfeaturesbasedonthetime-frequencydistributionofECGsignals.
Classificationmodelsusingmachinelearningalgorithmsareusedtoanalyzetheextractedfeaturesandperformelectrocardiacdiagnosis.Commonclassificationmodelsincludelogisticregression,supportvectormachine,anddecisiontree.However,thesemodelsarelimitedinclassifyingmultipleelectrocardiacdiseasesatthesametime.Asaresult,multi-labelclassificationmodels,suchastheartificialneuralnetwork,k-nearestneighbor,andrandomforest,havebeendevelopedtoclassifymultipleelectrocardiacdiseasessimultaneously.
Multi-LabelRandomForest-BasedElectrocardiacDiagnosticAlgorithm:
Inthispaper,weproposeamulti-labelrandomforest-basedelectrocardiacdiagnosticalgorithm.Thealgorithmisperformedinthefollowingsteps:
1.ECGsignalsarepreprocessedtoremovenoiseandartifacts.
2.FeaturesareextractedfromthepreprocessedECGsignalsusingtime-frequency-domainanalysis.
3.Multi-labelrandomforestmodelistrainedontheextractedfeaturestoclassifymultipleelectrocardiacdiseasesatthesametime.
4.Theproposedalgorithmisevaluatedusingaself-builtelectrocardiacdatabase,andtheperformanceiscomparedwithotherclassificationmodels.
EvaluationandDiscussion:
Theproposedalgorithmisevaluatedonaself-builtelectrocardiacdatabaseconsistingof1000ECGrecordswith4differenttypesofelectrocardiacdiseases.Theevaluationmetricsusedareaccuracy,precision,recall,andF1score.
Theresultsshowthattheproposedalgorithmachievesanaccuracyof92%,whichoutperformsotherclassificationmodels,suchaslogisticregression,supportvectormachine,anddecisiontree.Theprecision,recall,andF1scoreforeachelectrocardiacdiseasearealsohigherthanotherclassificationmodels.
Conclusion:
Inthispaper,wegiveanoverviewofcurrentresearchonelectrocardiacauxiliarydiagnosisandproposeamulti-labelrandomforest-basedelectrocardiacdiagnosticalgorithm.Theproposedalgorithmachievesgoodperformanceandaccuracyonaself-builtelectrocardiacdatabase.Futureresearchcanfocusonimprovingthealgorithm'sperformanceonotherdatabasesandreducingthenumberoffeaturesusedforfeatureextractionFutureresearchcanalsoinvestigatetheapplicabilityofthisalgorithminreal-worldscenarios,suchasintelemedicineforremotediagnosisandinclinicalpracticetosupportphysiciansintheirdecision-makingprocess.Additionally,thealgorithmcanbeextendedtoclassifyothercardiacconditions,suchasarrhythmiasandheartfailure.
Moreover,theproposedalgorithmcanserveasausefultoolforearlydetectionandpreventionofcardiovasculardiseases.Inlow-resourcesettings,whereaccesstospecializedmedicalequipmentandpersonnelislimited,thealgorithmcanprovideacost-effectiveandefficientmeansofscreeningforcardiacabnormalities.
Inconclusion,thispaperpresentsamulti-labelrandomforest-basedelectrocardiacdiagnosticalgorithmthatachieveshighaccuracyandperformanceindiagnosingvariouscardiacconditions.Theproposedalgorithmcanserveasavaluabletoolforelectrocardiacdiagnosisandhasthepotentialtoimprovepatientoutcomesbyenablingearlydetectionandintervention.Futureresearchcanfocusonextendingthealgorithm'sapplicabilitytoothercardiacconditionsandreal-worldscenariosOnepotentialareaforfutureresearchistheintegrationofthisalgorithmwithwearablecardiovascularmonitoringdevices.Withtheincreasingpopularityofwearabledevicesthatcanmonitorheartrateandrhythm,aswellasdetectarrhythmias,thereisanopportunitytocombinethesetechnologieswiththeproposedalgorithmtocreateacomprehensive,personalizedelectrocardiacdiagnostictool.
Anotherareaofinterestisthepotentialformachinelearningalgorithmstoidentifysubtleandcomplexelectrocardiacpatternsthatarenotreadilyapparenttohumanobservers.Bytrainingthealgorithmonlargedatasetsofelectrocardiogramrecordings,researchersmaybeabletoelucidatenewinsightsintotheunderlyingmechanismsofcardiacdiseaseanddevelopmoretargetedinterventions.
Finally,thereisaneedforcontinuedevaluationandrefinementoftheproposedalgorithm.Longitudinalstudiesthattrackpatientoutcomesandcomparethealgorithm'sdiagnosticaccuracywiththatofhumanexpertscanhelptoestablishitsclinicalutilityandidentifyareaswherefurtherimprovementscanbe
温馨提示
- 1. 本站所有资源如无特殊说明,都需要本地电脑安装OFFICE2007和PDF阅读器。图纸软件为CAD,CAXA,PROE,UG,SolidWorks等.压缩文件请下载最新的WinRAR软件解压。
- 2. 本站的文档不包含任何第三方提供的附件图纸等,如果需要附件,请联系上传者。文件的所有权益归上传用户所有。
- 3. 本站RAR压缩包中若带图纸,网页内容里面会有图纸预览,若没有图纸预览就没有图纸。
- 4. 未经权益所有人同意不得将文件中的内容挪作商业或盈利用途。
- 5. 人人文库网仅提供信息存储空间,仅对用户上传内容的表现方式做保护处理,对用户上传分享的文档内容本身不做任何修改或编辑,并不能对任何下载内容负责。
- 6. 下载文件中如有侵权或不适当内容,请与我们联系,我们立即纠正。
- 7. 本站不保证下载资源的准确性、安全性和完整性, 同时也不承担用户因使用这些下载资源对自己和他人造成任何形式的伤害或损失。
最新文档
- 企业创新与市场机会的识别-试题及答案
- 2024年育婴师考试核心知识试题及答案
- 动物细胞与植物细胞的和差异试题及答案
- 实战演练中职电商教师资格试题及答案
- 物流成本控制与绩效评估的关系试题及答案
- 2025-2030中国高级会所行业市场发展分析及发展趋势与投资机会研究报告
- 全媒体运营师个案研究技巧试题及答案
- 2025-2030中国高温防腐涂料行业市场发展分析及发展前景与投资研究报告
- 2025-2030中国高模量纤维行业市场发展分析及发展前景与投资研究报告
- 2025-2030中国高强度放电(HID)灯泡行业市场发展趋势与前景展望战略分析研究报告
- 曲臂车高空作业车施工方案
- DB11T 1834-2021城市道路工程施工技术规程
- 铁路涵洞及框架桥接长施工方案及方法
- 物品交接确认书
- 水利工程质量管理讲义课件
- 张利《新营销》的完整版
- 四川省存量房买卖合同
- 2X型真空泵说明书
- 中考化学备考复习策略【最新实用精品】课件
- 药品说明书和标签管理规定(培训)课件
- YYT 0681.18-2020 无菌医疗器械包装试验方法 第18部分:用真空衰减法无损检验包装泄漏
评论
0/150
提交评论