


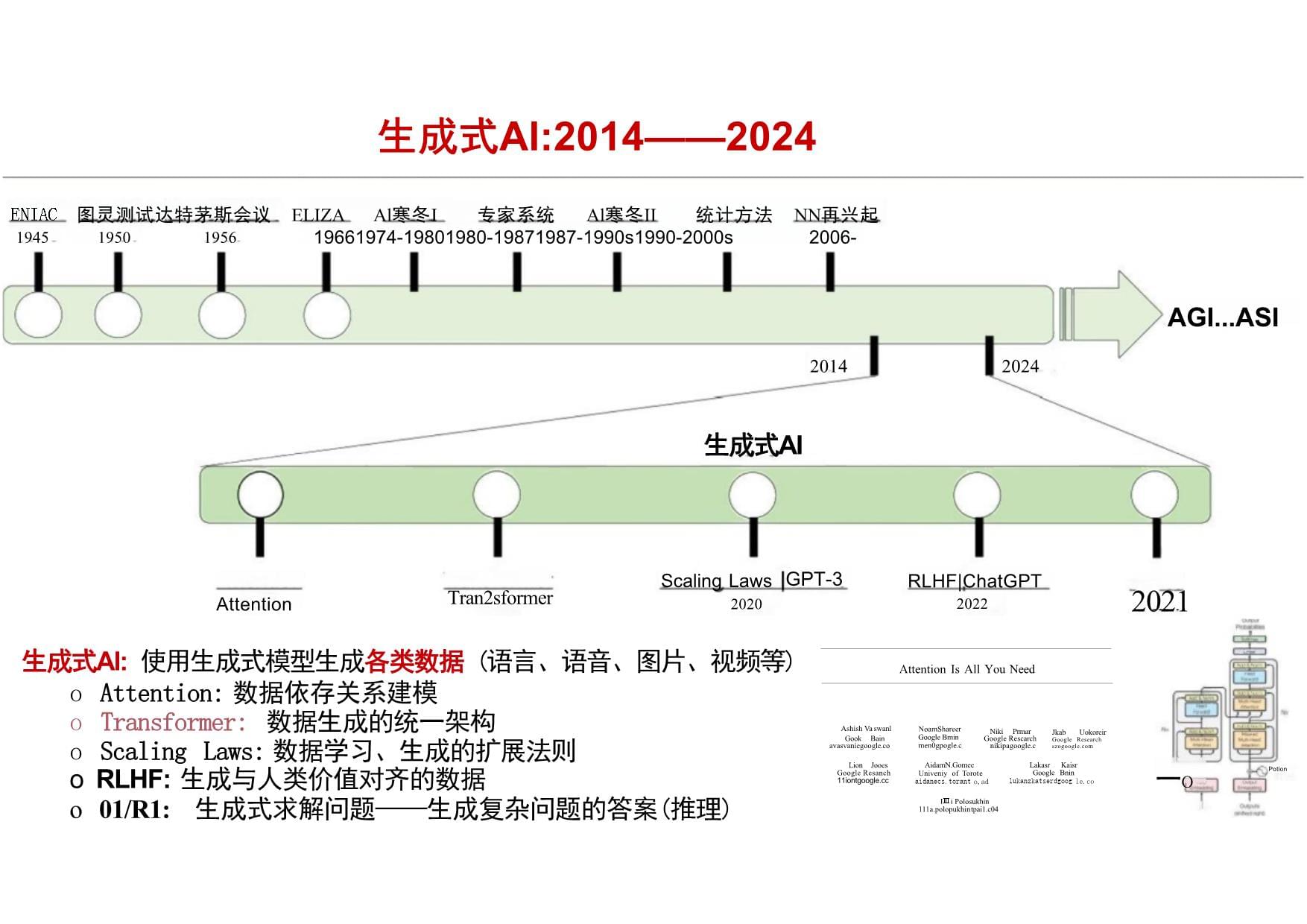

版权说明:本文档由用户提供并上传,收益归属内容提供方,若内容存在侵权,请进行举报或认领
文档简介
深度解读DeepSeek:
原理与效应熊德意天津大学dyxiong@https://dyxiong.github.iohttps://tjunlp-lab.github.io仁文伏羲
伏羲传语天津大学自然语言处理实验室The
Natural
Language
Processing
Laboratory
at
Tianjin
UniversityOpenEval营大语言模型发展路线图DeepSeek
V2-V3/R1技术原理
DeepSeek效应未来展望03报告目录生成式Al:2014——2024ENIAC
图灵测试达特茅斯会议ELIZA
Al
寒冬I专家系统Al
寒冬II
统计方法
NN
再兴起1945
1950
1956
19661974-19801980-19871987-1990s1990-2000s2006-AGI...ASI2014
2024生成式AI生成式AI:
使用生成式模型生成各类数据
(语言、语音、图片、视频等)o
Attention:
数据依存关系建模o
Transformer:
数据生成的统一架构o
Scaling
Laws:
数据学习、生成的扩展法则oRLHF:生成与人类价值对齐的数据o
01/R1:生成式求解问题——生成问题求解的过程和答案(推理)1414
Feature
Map1.hput
2.Convouional3.RNNmithatenion
4.Wordbymape
Feature
Eatraction
over
theimoge
Attention
Tran2sformerScalingLaws
|GPT-3
2020RLHF|ChatGPT2022Figure
L.Our
modellearmsawords/imagealignment.Thevisualized
attentional
maps(3)are
explained
in
Sctions3.1&5.42024water生成式Al:2014——2024ENIAC
图灵测试达特茅斯会议
ELIZA
Al寒冬I专家系统Al寒冬II
统计方法NN再兴起1945
1950
1956
19661974-19801980-19871987-1990s1990-2000s2006-AGI...ASI20142024生成式AI生成式Al:
使用生成式模型生成各类数据
(语言、语音、图片、视频等)o
Attention:
数据依存关系建模o
Transformer:
数据生成的统一架构o
Scaling
Laws:
数据学习、生成的扩展法则oRLHF:
生成与人类价值对齐的数据o
01/R1:
生成式求解问题——生成复杂问题的答案(推理)2021一ohaScaling
Laws
|GPT-3
2020RLHF|ChatGPT
2022Tran2sformerLakasr
KaisrGoogle
Bninlukanzkatserdgoogle.coAidamN.GomeeUniveniy
of
Torote
aidanecs.toranto,adAshishVaswanlGook
Bainavasvaniegoogle.coLion
Jooes
GoogleResanch
11iontgoogle.ccAttention
Is
All
You
NeedIⅢiPolosukhin111a.polopukhintpai1.c04Jkab
Uokoreir
Research
szogoogle.comNiki
PrmarGoogleRescarchnikipagoogle.cAttentionNoamShareerGoogleBmin
men0gpogle.cPotionBror0生成式Al:2014——2024ENIAC
图灵测试达特茅斯会议
ELIZAAl寒冬I专家系统Al寒冬II统计方法NN再兴起1945
1950
1956
19661974-19801980-19871987-1990s1990-2000s2006-AGI...ASI2014
2024生成式AI生成式Al:
使用生成式模型生成各类数据
(语言、语音、图片、视频等)o
Attention:
数据依存关系建模o
Transformer:
数据生成的统一架构o
Scaling
Laws:
数据学习、生成的扩展法则oRLHF:生成与人类价值对齐的数据o
01/R1:生成式求解问题——生成复杂问题的答案(推理)Attention
Tran2sformerScaling
Laws|GPT-3
2020RLHF|ChatGPT
2022ComputePF-days.non-ermbedding2024Dataset
Size
tokensParametersnon-embeddingTastLoss图灵测试达特茅斯会议ELIZA
Al寒冬I专家系统
Al寒冬II
统计方法NN
再兴起E1945
1950
1956
19661974-19801980-19871987-1990s1990-2000s2006-20142024生成式AI生成式Al:使用生成式模型生成各类数据
(语言、语音、图片、视频等)o
Attention:数据依存关系建模o
Transformer:
数据生成的统一架构o
Scaling
Laws:
数据学习、生成的扩展法则o
RLHF:
生成与人类价值对齐的数据o
01/R1:生成式求解问题——生成复杂问题的答案(推理)Scaling
Laws|GPT-3RLHF|ChatGPT
01/R12020
2022
2024生成式AI:2014——2024andtaaagersd
poky
tanrrd
medetrertooenerlearinyadooithmTran2sformerAGI...ASIAtte01tion生成式Al:2014——2024ENIAC
图灵测试达特茅斯会议
ELIZAAl寒冬I专家系统Al寒冬II统计方法NN再兴起1945
1950
1956
19661974-19801980-19871987-1990s1990-2000s2006-AGI...ASI20142024生成式AI生成式Al:使用生成式模型生成各类数据
(语言、语音、图片、视频等)o
Attention:
数据依存关系建模o
Transformer:
数据生成的统一架构o
Scaling
Laws:
数据学习、生成的扩展法则oRLHF:生成与人类价值对齐的数据o01/R1:生成式求解问题——生成复杂问题的答案(推理)Questiom:Ifa>1,thenthesumof
thereal
solutions
of
va-va+x=xis
equal
toResponse
<think>Tosolvethe
equationva-Va+x=x,let's
start
by
squaring
both…
(va-Va+x)=x²
一a-va+x=x²Rearrangetoisolatethe
innersquare
root
terme(a-x²)²=a+x一a²
-2ax²+(x²)²=a+x一x⁴-2ax²
-x+(a²
-a)=0…Wait,wait.WaitThat'sanahamoment
Ican
flag
hereLet'sreevaluatethisstepby-step
to
identify
if
the
correct
sum
can
be
Westartedwith
the
equatioreVa-va+x=xFirst,let'ssquare
both
sidesa
-
V
a
+
x
=
x
²
一
V
a
+
x
=
a
-
x
²Next,Icouldsquareboth
sides
again,treating
the
equation:…Attention
Tran2sformerScaling
Laws
GPT-3
2020RLHF|ChatGPT
20222024Trainedtopredictthenext
word
in
a
sentence:Thecat
ischasing
the10010100101010
100101001100101001自然语言处理:人类语言的智能化
处理与分析,使计算机具备听、说、
读、写、译等人所具备的语言能力语言模型:自然语言统计建模,简
单说,就是预测句子中的下一个单
词是什么自然语言处理与语言模型NATURALLANGUAGE
PROCESSING(NLP)
FORARTIFICIALINTELLIGENCEdog
5%mouse70%
squirel
20%
boy
5%house
0%Language
ModelsThikpadmAnthropicAlWebGPTSErnie3.0TitanGopherGLaMBLOOMmTOBLOOMZ
养Galatica
XTO
排
9-10-NAVER11-12\InstructGPTCodeGenMT-NLGOPTGPT-NeoX-20BTk-InstructCohereWeLM
CmTII)wssHUAWEIYuLan-ChatStarCoderCodeGen2ChatGLMFalconPaLM2PythiaVicunaPanGu-ZInternLME2
QwenMistralE2Qwen2DeepSeek-V2XLLaMA3大语言模型:2018——2024OPT-IMLXZhao
et
al.A
Survey
of
Large
Language
Models.arXiv:2303.18223inspur
Yuan
1.0GGY4-6Bard0LLaMA周DeepseekMixtralGMiniCPMGemmaOGG(xSparrowFlan-T5Flan-PaLMLuminousNLLBAl21labs3AAIErnie
3.0Jurassic-1CPM-216
7-12-—
—2024—1-6→GPT-4身
0
LLaMA2PanGu-αHUAWEE2PLUGG
FLANGPT-3身Codex
窃
-
5-8CodeGeeXGLM
AlexaTM智谱·面aG
mT51-4GO◎UL2PaLMYaLM-
2023ChatGPT
身—2019—2020
2021G
T5
GGShardAlphaCodeChinchilla百源开照LaMDAPublicly
Available2022I1-3HyperCLOVA7-1011-12.Ai2X对齐训练数据PromptRosponses软件资源分配docker
kubernetes任务调度模型训练预训练
对齐训练
SFTRLHFDPOBet
of
N
sam
plingData
Parallel
TensorParallelExpert
Parallel
ZeROPipelineParallelSoquene
ParalelFashAttention数据处理和管理Data
Processing
and
Managem
ent算力管理Com
puting
Managem
ent模型评测OpenEva
UltraEval
OpenCompassXChatbot
ArenaFlagEval
-
openLMLaderbard知识能力价值对齐负载均街性能监控安全可信专业领域弹性扩展容错机制大语言模型:技术栈通用模型行业模型Specialized
Model行业模型领域对齐训练动态批处理模型量化模型剪枝算子优化模型蒸馏性能监控处理流程数据去重领域分类910B910A质量筛选版本控制slurm通用模型General-purposeModel预训练数据数据分类行业对齐数据领域微调训练应用层Application行业棋型部署
行业模型评测网页
书籍
代码
论文百科品AAMD语营检测内容过滤M1350M1300模
型
部
着自主规划图文创作智能客服信息检索H100A100工具调用代码生成nVIDIA.评测数据行业数据硬件Ascond数据处理
预训练
后训练
应用部署数据治理
基座模型
对齐模型
红队测试数据要素
自监督学习
■
微调&强化
·商业落地知识源头
能力涌现
安全可信
模型压缩o
训练范式
o关键·预训练——基座模型
●模型架构·后训练——对齐模型
·训练算法·推理训练——推理模型·
扩展法则杀手锏:性能/成本曲线|性价比大语言模型:生命周期与范式The
bitter
lesson
is
based
on
the
histor-ical
observations
that1)Al
researchers
have
often
tried
to
build
knowledge
intotheir
agents,2)this
always
helps
in
the
short
term,and
is
personally
satisfying
to
the
researcher,but
3)in
the
longrun
it
plateaus
and
even
inhibits
furtherprogress,and
4)breakthrough
progresseventually
arrives
by
an
opposing
ap-
proach
based
on
scaling
computation
bysearch
and
learning.扩展法则The
BitterLessonSasha
Rushand
Daniel
Ritter.SpeculationsonTest-TimeScaling.2024[Sutton,2019]Two
Era'sAlignment
PipelinesHumanInstructions(-10k)
PPO
optinizationBase
Model
SFT
Model
AlignedModel成本较低大部分实验室可做成本高昂(上千万)
少数企业/实验室可做NewSynthetieCompletionsxNroundsDPQ,PPORejoction
Samplng.ormutpeoptintzations
Aligned
ModelN+1-
Final
Model大语言模型:后训练范式Aligned
ModelNHumanpreferences(~1M*?)Re-usepreferencepromptsReward
ModelBase
ModelInfercornectHuman+SyntheticInstructions(-1M+7initiatFTtrahingReward
Model/LLMJudge~Llama
3.1/Nemotron~InstructGPTHumanpreferencs(-100k)Re-use
preference
promptsSOpenAIQ*exp
d)Thederoninalorofafactonis7hasthan
3ines
she
munnetactlt4⑧
●urcaltenumr回四④
som
dinaler
is-7.-5eGPTain(l①
wothasme7=25①
a
7四②
x
-14●@
sox-7过程奖励模型PRMReminder:AlphaZero推理语言模型?Sasha
Rushand
Daniel
Ritter.Speculations
on
Test-TimeScaling.2024MCTS大语言模型发展路线图DeepSeek
V2-V3/R1技术原理DeepSeek效应未来展望03报告目录2023.11DeepSeek
V12024.5DeepSeek
V2天边的两多云(国内外现状)o模型架构:大部分企业采用已验证架构(试错成本高昂)【
不
敢
】o推理模型:大部分实验室仍在苦苦猜测摸索Q*/01(OpenAl保密)【
不
知
】DeepSeek:2023——2024.11DeepSeek
R1-Lite2025.01DeepSeek
R12024.12DeepSeek
V3DeepSeek
V2主要创新o
DeepSeekMoEo
MLADeepSeekMoEo
稀疏激活:计算不随规模呈线性增长o
相比传统MoE:细粒度专家(共享+路由)o
路由&通信改造:·
Device-Limited
Routing·Auxiliary
Loss
for
Load
BalanceToken-Dropping
StrategyTransformerBlock×LFeed-Forward
NetworkRMS
NormAttentionDeepSeekMoEOOOO
OO00
Routed
ExpertOutput
Hidden
h{Shared
Expert1
N₅
1
2
3
4
N-1
N,Router
dhl
Top-KInput
Hidden
utMulti-Head
LatentAttention(MLA)OCached
During
InferenceOutput
Hidden
u:OOOOOOO0Multi-HeadAttentionOO
0OLatent
c8LatentcOV2规模:236B
totalparameters,21B
activatedparameters,128K
context
window
Input
Hidden
heOOOO
OO00[lqS;a&concatenatelafapply
RoPE{[kS,;k{](
ooOconcatenatelkE回applyRoPEDeepSeek:
技术创新——模型架构|V2rocrhKeyscompresed
LatentKVQueriesMLA:低秩压缩,降低KVcache占用空间ached
During
InferenceRMS
NormMuHeadAttention(MHA)!Grouped-queryAtention(GOA)!Mut-queryAttenton(MOA!MuuHeadLatentAttenton(MLA){k
匠{qS3OCoO{vH·Mixtral8x7BCommand
RLLaMA38BO
LLaMA
234BMistral
7BLLaMA133BLLaMA
213BLLaMA
270BO
LLaMA165BLLaMA
1
Family-LLaMA2FamilyLLaMA3
FamilyMixtral
FamilyCommand
R
FamilyQwen1.5
Family(a)
(b)杀手锏:性能/成本曲线|性价比KVCacheforGeneration(KB/Token)DeepSeek67Breducing
KV
cache
by93.3%100200300400MaximumGenerationThroughput(Tokens/Sec)DeepSeek67BDeepSeek-V20TrainingCosts(KGPU
Hours/T
Tokens)DeepSeek67Bsaving
42.5%oftrainingcosts050
100150DeepSeek-V2Mixtral8x22B
LLaMA
370BCommand
R+DBRXQwen1.572B
ODeepSeek:
技术创新——模型架构|V2训练开销存储开销生成速度0
20
40
60
80
100ActivatedParameters(Billions)Performance
(MMLU)807570656055576%of
maximum
throughputDeepSeek
67BGrok-1DeepSeek-V2DeepSeek-V2Qwen1.532B01
N₅
1
2
3
4N--
1N,Router
dhhlTop-KOO0O
◎
InputHidden
uMulti-Head
Latent
Attention(MLA)OOcachedDuring
InferenceOutput
Hidden
u:
OOOO
OOO0Multi-Head
Attention{[qS,;ql}
O0O
{[k{,;k{J}[concatenatef
conctenotelDeepSeek
V3主要创新o
InfrastructuresoMulti-Token
Prediction
(MTP)Infrastructureso减少流水线气泡o
高效节点间All-to-All通信o
FP8训练o
低精度存储与通信MTP:
一次预测多个topkenTransformerBlock
×LFeed-ForwardNetworkRMS
NormAttentionRMS
NormetMin
MaddouputHeoad-4MTPModue1oupurHeadfranfomerliockMTP
Modube2etoupgHeadTransformerlocktrngfrmerlock×LlherhaFmbeddlguebwr
mtDeepSeek:
技术创新——模型架构|V3V3规模:671Btotalparameters,37Bactivatedparameters,trainedon14.8TtokensImput
Hiden
h,Oo00'o000OO-0Olatent
c{
LatentcODeepSeekMoEOutput
Hidden
h{93
(qRouted
Expert
Shared
Expertkf|
回applyRoPE后fapplyRoPE{k
匠OO{v&B杀手锏:性能/成本曲线|性价比DeepSeek:
技术创新——模型架构|V3MMLU
Redux
ZeroEval
Score
VS
Input
API
Price($/1M
Tokens)Training
CostsPre-Training
Context
Extension
Post-TrainingTotalin
H800
GPU
Hours2664K119K5K2788Kin
USD$5.328M$0.238M$0.01M$5.576MTable
1|Training
costs
of
DeepSeek-V3,assumingtherentalprice
of
H800
is
$2per
GPUhour.Duringthe
pre-trainingstate,training
DeepSeek-V3oneachtrilliontokens
requiresonly
180K
H800
GPU
hours,i.e.,
3.7daysonourownclusterwith2048
H800GPUs.Consequently,our
pre-training
stageiscompleted
in
less>E.g.Llama3405B
used30.8M
GPU-hours,while
DeepSeek-V3looksto
be
a
stronger
model
at
only
2.8M
GPU-hours(~11X
less
compute).Super
interesting!And
DeepSeek
was
trained
in
H800's
which
areprobably
also
a
tad
(or
noticeably?)slower
than
Meta's
H100's.大规模高性能加速器
(折旧)大模型研发人员成本大模型架构技术探索成本
大模型数据成本大模型最终训练成本DeepSeek:
技术创新——模型架构|V3
成本杀手锏:性能/成本曲线|性价比thantwomonthsandcosts2664K
GPU
hours.SebastianRaschka@rasbt大模型部署推理成本大模型研发成本成本-冈DeepSeek
V2-V3及R1在模型架构上选择稀疏MoE模型而非稠密模型,并进行和积累了大量技术创新,包括MLA、FP8
训练、MoE
All-to-AlI通信瓶颈解决、MTP等,
这些技术并不是所有都是原始创新,但是能够进行如此多大模型架构底层创新的实验室,在全世界可能也只有少数几个;DeepSeek
所有模型架构上的创新均是围绕“降本增效”:在基本不损害性能前提
下,尽可能通过算法挖掘和提升硬件训练和解码效率美国采取芯片禁令(全球三级管控)策略维持自己的Al领导地位,DeepSeek
算法绕过了美国的算力护城河DeepSeek:
技术创新——创新程度DeepSeek
R1主要创新o
DeepSeek-R1-Zero:
大规模RL
训练,发现了RL
训练的Scaling
Laws,RL训练涌现“aha”时刻o
推理模型训练技术框架:
4步法,有效解决了R1-Zero
存在问题,将推理与对齐合为一体o
强化学习训练框架:GRPO,
来
自DeepSeekMath,降低了强化学习训练成本o
推理模型蒸馏:
将大模型推理能力蒸馏到小模型,优于小模型直接进行推理训练(规模效应)为什么MCTS+PRM是“误区”o
The
bitter
lesson:scalabilityo
OpenAl
竞争策略DeepSeek:
技术创新——推理模型|R1DeepSeek:
技术创新——推理模型|R1-ZeroLarge-scale
Reasoning-OrientedReinforcement
Learning3.通过prompt
策略引导模型思考和给出答案,避免基座
模型不能生成停止符使用标记<think></think><answer></answer>R1-Zero存在问题:poorreadability,language
mixingstepsDeepSeek-v3-Base
DeepSeek-R1-Zero2.RL
Training
Scaling
Law:
涌现reflection
、aha自动涌现出搜索、反思、顿悟、纠错与testing-time
scaling
law—致,可从性能增长曲线和长
度增长曲线推出推理时scaling
lawA
conversation
between
User
and
Assistant.The
user
asks
a
question,and
the
Assistant
solves
it.The
assistant
firstthinks
aboutthereasoningprocess
inthe
mind
and
then
provides
the
userwith
the
answer.The
reasoning
process
and
answer
are
enclosed
within
<think></think>and<answer></answer>tags,respectively,i.e,<think>reasoningprocesshere</think><answer>answerhere</answer>.User:prompt.Assistant:Table1|TemplateforDeepSeek-R1-Zero.promptwillbe
replaced
with
the
specific
reasoningquestion
during
training.1.强化学习训练规模大业内通常训练几十RL
steps,DeepSeek训练几千RL
Tülu
3最大发布模型只训练了~50RL
stepsKerconnects.ai/p/deepseek-r1-recipe-for-01Fgure3/The
average
rspone
lknghfDwpskRI-Zme
onthe
trainingstduring
theRLpoces.DepSok-R1-ZronuhuralylearstosbvereasoningtaskswihmarethinkngtimeFgum2|AIMEaecurayafDwpskRI-ZcmduringtrainingForeachquotbnwsmple16responsesandakuletheowrallawerageaccuncytoensure
astulleevaluation.stepsDeepSeek-V3-base(200K
samples)Step3.
RejectionSamplingSFT
3/4reasoning
data(600K)1/4
general
instruction
data
(200K)Reasoning
Data长CoT
数据General-Purpose
ModelDeepSeek-R1Step
0.GeneratingLong
CoT
data
Step
4.General
RLRLHF
Preference
Tuning
with
safety
rewardso
DeepSeek-R1
不是唯一的推理模型框架,2025年将出现更多新的框架o
要复现上述框架,需要DeepSeek
开源相关数据Step
2.Reasoning-orientedRLStep3
Reasoning
Data
类似训练R1-Zero
Math,Code,Logic直至训练收敛
(600K
samples)Few-shot
ICL+
人工后期refining
Reasoning
RL
with
rule-based
rewardsDeepSeek:
技术创新——推理模型|R1
Recipe大规模强化学习DeepSeek-R1-Zero
中间推理模型Step
3
Instruction
DataWriting,QA,trans,etc.SFTCheckpoint
RL-tuned
ModelStep1.
ReasoningSFT
Cold
Start1.强化学习框架GRPO(DeepSeekMath)采用蒙特卡洛采用估算以取代Value模型,降低
计算和存储开销2.强化学习奖励模型o
采用easily
verifiable
rewards rewardo
避免过程奖励模型:计算复杂,容易reward●·AccuracyReferenceModelRewardModelqTrained
ModelsFrozenModelsA₁A₂AFigure4|DemonstrationofPPOandourGRPO.GRPOforegoesthevaluemodel,insteadestimatingthebaselinefromgroupscores,significantlyreducingtrainingresources.hackingDeepSeek:
技术创新——推理模型|RLFormat
reward·Language-consistency
rewardKLReference
ModelReward
ModelGroupComputationGAE
APolicyModelPolicyModelValue
Model0₁0₂0GRPO◆田-
rPPOr1T₂|KLrgqV0Qwen2.5-Math-1.5B,SFTQwen2.5-14B,Qwen2.5-32B,Llama-3.1-8B,andStep
3
Reasoning
DataMath,Code,Logic(600K
samples)Step
3
Instruction
DataWriting,QA,trans,etc.(200K
samples)推理模型蒸馏到小模型o
reasoning能力可以蒸馏到小模型o
大模型蒸馏到小模型优于小模型直接通过大规模RL训练o
再次验证了模型规模在AGI发展中的重要性o推理者同样需要规模支撑DeepSeek:
技术创新——推理模型|推理能力蒸馏DeepSeek-R1-Distill-Qwen2.5DeepSeek-R1-Distill-LlamaLlama-3.3-70B-InstructQwen2.5-Math-7B,DeepSeekvs
OpenAICreated
by
pc■openAl-o1-1217MMLUDlamond囊uygnSource:DeepSeek
OHiclar
Website
Morecharts:杀手锏:性能/成本曲线|性价比DeepSeek:
技术创新——推理模型|R1Pricing:InputandOutput
PricesUSD
per
1MTokens■Input
price
■Output
pricecodstorceAcompectve
erooramming
plottormshere
coders
solheMATH-500AcolecticnAIME2024Amothor500
toughmothprobemssWE-bench
VerifiedDeepSeek-R¹ondreosonlngAtest
ofGPOAModelsLogicalLevel
1Level
2Level
3OpenSource?Model
SizeDeepSeek-R1(API)76.10%90.48%77.14%61.70%Yes671BDeepSeck-R1(网页)74.84%80.95%78.57%63.83%Yes671Bol-preview72.33%88.10%74.29%55.32%NoundisclosedDeepSeek-R1(非官方API-together)70.44%80.95%78.57%48.94%Yes671BQwQ-32B63.52%73.81%70.00%44.68%Yes32Bhunyuan-turbo-latest62.26%85.71%65.71%36.17%NoundisclosedGLM-Zero-preview61.64%71.43%71.43%38.30%NoundisclosedDoubao-pro-32k61.01%83.33%62.86%38.30%NoundisclosedYi-Lightning52.83%64.29%60.00%31.91%NoundisclosedDeepSeek-V2.5-121049.69%69.05%57.14%21.28%YesundisclosedErnie-4.0-Turbo-8k49.06%66.67%54.29%25.53%NoundisclosedDeepSeek-V349.06%66.67%52.86%27.66%Yes671BSenseChat-5-120247.17%64.29%50.00%27.66%NoundisclosedGPT-4-Turbo42.77%57.14%48.57%21.28%NoundisclosedSpark4.0Ultra39.62%57.14%44.29%17.02%NoundisclosedMoonshot-v1-32k38.99%45.24%48.57%19.15%NoundisclosedGPT-3.5-Turbo29.56%35.71%35.71%14.89%NoundisclosedDeepSeek-R1(网页)平均思考时间Average
Times(s)AllCorrectWrongOverall147.26100.69285.83Level
183.5763.88167.25Level
2132.4991.98281.00Level3226.19158.37345.88DeepSeek:
技术创新——推理模型|R1TJUNLP实测DeepSeek-R1逻辑推理性能DeepSeek
R1是在探明方向
(OpenAl
o1引领和证实的方向)上进行0-1的创新突破,独立探索出基于大规模强化学习的大语言模型推理技术路线,避开了过去一年
多(
自OpenAl
的Q*
在社交媒体讨论)业内广泛思索的通过在训练中进行显式搜索、
过程奖励模型(即Search+PRM)
实现推理的“误区”;贡
献
:o独立探索出推理技术路线o将技术路线公开发布(解惑了业内的“不知”)o模型开源
(MITLicense)Dee
pSeek
R1打破了美国第一梯队企业以闭源形成的技术护城河,进一步动摇
了美国的"AIDominance"DeepSeek:
技术创新——创新程度大语言模型发展路线图DeepSeek
V2-V3/R1技术原理DeepSeek
效应未来展望报告目录Overnight,Microsoft,NVIDIA,andAmazonallconnectedtoDeepSeek!
Andrew
Ng:Al
inChina
isonthe
rise.New
InteligenoeSouree·Jan3116:49
文/AMSFT+0.35%
NVDA+1.71%AMZN
+195%
口unusual_whales@unusualwhales·Jan28BREAKING:Thisisnotamemecoin.Thisis
Nvidia,SNVDA,themostvaluablecompanyin
the
word
before
today.t
isdown
17%.It
lost$560
billion
in
marketcaptodaysofar,thelargest
in
market
history.<AppsTop
Charts
All
AppsFree
Apps
Paid
AppsMicrosoft,NVIDIA,andAmazonembraceDeepSeekR1,alongwithUSACloud
Computingplatforms.Andrew
NgandtheformerCEOofIntelpraise
DeepSeek's
innovativecapabilities.开源vs
闭
源创新&人才&Vision2
ChatGPTThe
official
app
by
OpenAI3
ThreadsConnect
andshare
ideasDeepSeek:
效应OnthelastdayofJanuary,theenthusiasmfrom
DeepSeekshows
nosigns
of
waning.算力价格战认知误区1
DeepSeek-AlAssistantIntelligent
AIAssistantOpenme17so.00s2.00s₄.00S₆.00s₈.00$10.00$12.00
$14.00
$16.00
$18.00
S20.00
$22.00
$24.00
S26.00
S28.00
$30.00Price(USD
per
MTokens)产品:性价比永远是王道
技术也是如此
数百亿美元构建的前沿技术护城河一夜间被攻破DeepSeek
R1DeepSeek
V3Gemini1.5
Pro(Sep)
Qwen2.5
MaxLlama
3/370B
01-minio3-mini
Claude
3.5
Sonnet
(Oct)GPT-40(Nov'24)Mistral
Large
2(Nov
24)DeepSeek:
效应——算力价格战AArtificial
Analysis015550-Llama
3.18B95-90-85-80-75-70-65-60-ArtificialAnalysisQualityIndexGPT-3
选择闭源之后,大模型开源vs
闭源之争、之战一直存在DeepSeek
R1的开源发布,一举赶超闭源大模型,是大模型开源史上的里程碑美国Al第一梯队企业的前沿技术封闭被打破开源vs
闭源不仅涉及技术的公开性,也关乎Al安全治理Thiscoderepositoryandthemodelweightsare
licensed
underthe
MIT
License.DeepSeek-R1series
supportcommercialuse,allowforanymodificationsandderivativeworks,including,butnotlimitedto,distillationfor
trainingotherLLMs.Pleasenote
that:·DeepSeek-R1-Distill-Qwen-1.5B,DeepSeek-R1-Distll-Qwen-7B,DeepSeek-R1-Distill-Qwen-14BandDeepSeek-R1-Distil-Qwen-32BarederivedfromQwen-2.5series,whichareoriginallylicensedunderApache2.0
License,andnowfinetunedwith800ksamplescuratedwith
DeepSeek-R1.·DeepSeek-R1-Distll-Llama-8BisderivedfromLlama3.1-8B-Baseandis
originally
licensed
under
llama3.1
license.·DeepSeek-R1-Distll-Llama-70BisderivedfromLlama3.3-70B-Instructandis
originally
licensed
underlama3.3license.samaltmancO-HOST
·
4dagoyes,wearediscussing.ipersonallythinkwe
have
been
on
the
wrong
side
of
history
here
and
need
tofigureouta
different
open
source
strategy;not
everyone
at
openai
shares
this
view,and
it's
also
notourcurrenthighest
priority.白个
5
1
0
凸
2Share
…DeepSeek:
效应——开源
vs
闭源lolzinventor
·5d
agoWould
you
consider
releasing
some
model
weights,and
publishing
some
research?曰
164Award
Share
…OpenAI
CEO
Sam
Altman
IVerified7.LicenseDeepSeek:
效应——认知误区如果ChatGPT刷新了我们对Al的认知,那么DeepSeek在某种程度上颠覆了:o
美国人对中国Al水平的认知:长久以来,美国认为中国在Al科技创新上更多是跟随者角色o
大模型研发成本的认知:大模型研发成本需要数千万乃至上亿美元14.中
国
版
的Sora
模型何时到来,可以看中国
版
的ChatGPT
何时到来。过去
一年,国内
大语言模型发展迅速,甚至出现了百模大战
的热闹景象,但“热闹”较多的是同质化竞
争,较少的是底层基础技术的原创性突破。15.
国内和国外大模型的差距不在于模型能
力高低,也不在于应用,而在于底层核心技
术
。而底层核心技术突破的最主要障碍不是
算力受限,也不是数据规模和质量受限,而
是缺乏足够数量的具有技术远见、敢于技术
冒险的大模型人才。16
.
大模型技术仍然在不断发展和突破中,
未来格局存在很多变数。大模型顶尖人才技术型人才:锐意进行大模型底层技术创
新和冒险(第
一
类人才)战略型人才
:具有AGI技术远见和vision
(第二类人才)为巩固并提升我国在这
一
领域的
国际竞争力,可以从以下布局和规划
着手。第
一
,进
一
步提升以大模型为
代表的前沿人工智能在国家科技和产
业发展中的战略地位,成立人工智能
工
作
小
组
,
领
导AI
产
研
咨
询
委
员
会
,
统筹资源,制定AI
政策和计划,推进
人
工
智
能
技
术
创
新
和
产
业
发
展。
第
二,重点规划和建设前沿人工智能相
关的国家基础设施,包括超级智算网
络、通用及行业数据基础设施、大规
模人工智能软件基础平台、人工智能
安全与测评基础设施、大模型开源平
台等。第三,开展大模型关键理论和
技术攻关,啃硬骨头,探新疆域,研
发
经
得
起
实
践
考
验
的
硬
核
技
术
。
第
四,
培
育
和
建
立
大
模
型
创
新
发
展
生
态,形成大模型技术创新氛围,鼓励
耐心资本敢投广投大模型硬核技术创
业企业。第五,重视人工智能人才培
养和成长,培养
一
批具有长远眼光
温馨提示
- 1. 本站所有资源如无特殊说明,都需要本地电脑安装OFFICE2007和PDF阅读器。图纸软件为CAD,CAXA,PROE,UG,SolidWorks等.压缩文件请下载最新的WinRAR软件解压。
- 2. 本站的文档不包含任何第三方提供的附件图纸等,如果需要附件,请联系上传者。文件的所有权益归上传用户所有。
- 3. 本站RAR压缩包中若带图纸,网页内容里面会有图纸预览,若没有图纸预览就没有图纸。
- 4. 未经权益所有人同意不得将文件中的内容挪作商业或盈利用途。
- 5. 人人文库网仅提供信息存储空间,仅对用户上传内容的表现方式做保护处理,对用户上传分享的文档内容本身不做任何修改或编辑,并不能对任何下载内容负责。
- 6. 下载文件中如有侵权或不适当内容,请与我们联系,我们立即纠正。
- 7. 本站不保证下载资源的准确性、安全性和完整性, 同时也不承担用户因使用这些下载资源对自己和他人造成任何形式的伤害或损失。
最新文档
- 人防车位流转合同范例
- 七年级下册数学教案【6篇】
- 会会议合同标准文本
- 以艺术促进学生情感表达能力计划
- 会与活动公司合同标准文本
- 个人校车出租合同标准文本
- 2025建筑工程合同封面
- 2025建筑幕墙施工合同
- 供货肉类合同标准文本
- 幼儿园专题讨论教学方案计划
- 黄金卷02(广州专用)-【赢在中考·黄金预测卷】2025年中考数学模拟卷(考试版)
- 2025-2030年班用帐篷项目投资价值分析报告
- 生物会考试题及答案
- 2025年合肥二模数学试题及答案
- 血管活性药物静脉输注护理解读
- (一模)赣州市2025年高三年级摸底考试地理试卷(含答案详解)
- 2025届武汉市二调数学质量分析正式版【课件】
- 2024-2024年上海市高考英语试题及答案
- 电力各种材料重量表总
- 08SS523建筑小区塑料排水检查井
- 正比例函数和反比例函数专项复习试题
评论
0/150
提交评论