
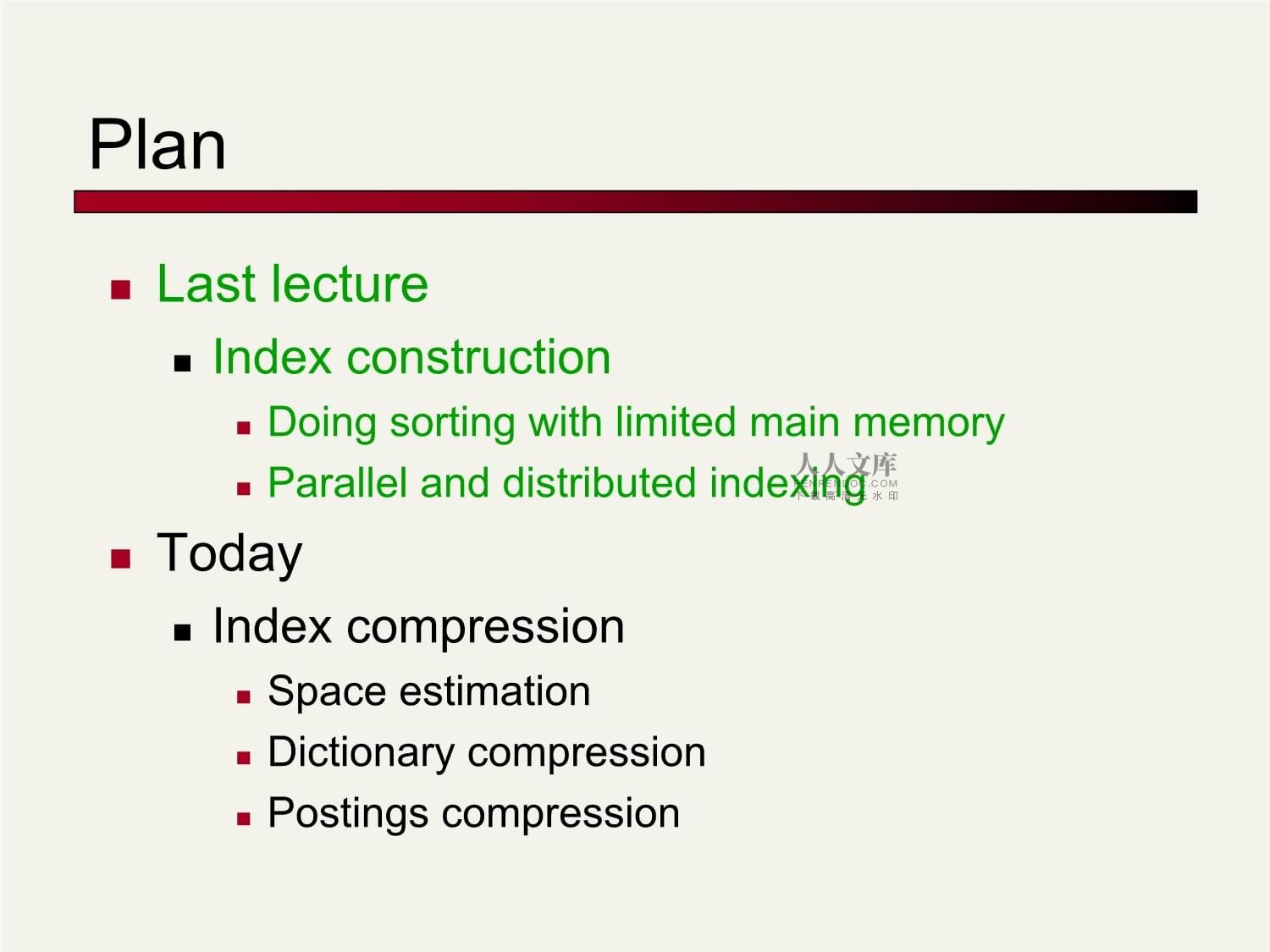



版权说明:本文档由用户提供并上传,收益归属内容提供方,若内容存在侵权,请进行举报或认领
文档简介
1、An Introduction to IR5th CourseChapter 5:Index compressionPlanLast lectureIndex constructionDoing sorting with limited main memoryParallel and distributed indexingTodayIndex compressionSpace estimationDictionary compressionPostings compressionCorpus size for estimatesConsider N = 1M documents, each
2、with about L=1K terms.Avg 6 bytes/term incl. spaces/punctuation 6GB of data.Say there are m = 500K distinct terms among these.Recall: Dont build the matrixA 500K x 1M matrix has half-a-trillion 0s and 1s.But it has no more than one billion 1s.matrix is extremely sparse.So we devised the inverted ind
3、exDevised query processing for itWhere do we pay in storage?Where do we pay in storage? PointersTermsIndex sizeStemming/case folding/no numbers cutsnumber of terms by 35%number of non-positional postings by 10-20%Stop wordsRule of 30: 30 words account for 30% of all term occurrences in written text
4、= # positional postings Eliminating 150 commonest terms from index will reduce non-positional postings 30% without considering compressionWith compression, you save 10%Storage analysisFirst, we will consider space for postingsBasic Boolean index onlyNo analysis for positional indexes, etc.We will de
5、vise compression schemesThen we will do the same for the dictionaryPositional index compression for advanced studyPostings: two conflicting forcesA term like Calpurnia occurs in maybe one doc out of a million we would like to store this posting using log2 1M 20 bits.A term like the occurs in virtual
6、ly every doc, so 20 bits/posting is too expensive.Prefer 0/1 bitmap vector in this case Postings file entryWe store the list of docs containing a term in increasing order of docID.Brutus: 33,47,154,159,202 Consequence: it suffices to store gaps.33,14,107,5,43 Hope: most gaps can be encoded with far
7、fewer than 20 bits.Variable length encodingAim:For Calpurnia, we will use 20 bits/gap entry.For the, we will use 1 bit/gap entry.If the average gap for a term is G, we want to use log2G bits/gap entry.Key challenge: encode every integer (gap) with as few bits as needed for that integer.Variable leng
8、th codes achieve this by using short codes for small numbers(Elias) g codes for gap encodingRepresent a gap G as the pair length is log2G in unary and uses log2G +1 bits to specify the length of the binary encoding of the offsetoffset = G 2log2G in binary encoded in log2G bits.Recall that the unary
9、encoding of x isa sequence of x 1s followed by a 0.g codes for gap encodinge.g., 9 is represented as .2 is represented as .Exercise: what is the g code for 1?Exercise: does zero have a g code?Encoding G takes 2 log2G +1 bits.g codes are always of odd length.ExerciseGiven the following sequence of g-
10、coded gaps, reconstruct the postings sequence:1110001110101011111101101111011From these g-decode and reconstruct gaps,then full postings. What weve just doneEncoded each gap as tightly as possible, to within a factor of 2.For better tuning and a simple analysis we need a handle on the distribution o
11、f gap values.Zipfs lawThe kth most frequent term has frequency proportional to 1/k.freq. = c / rank,1We use this for a crude analysis of the space used by our postings file pointers.Not yet ready for analysis of dictionary space.Zipfs law log-log plotRough analysis based on ZipfThe i th most frequen
12、t term has frequency proportional to 1/iLet this frequency be c/i.LetThe k th Harmonic number isThus c = 1/Hm , which is 1/ln m = 1/ln(500k) 1/13. So the i th most frequent term has frequency roughly 1/13i.Postings analysis contd.Expected number of occurrences of the i th most frequent term in a doc
13、 of length L is:Lc/i L/13i 76/i for L=1000.Let J = Lc 76.Then the J most frequent terms are likely to occur in every document.Now imagine the term-document incidence matrix with rows sorted in decreasing order of term frequency:Rows by decreasing frequencyN docsmtermsJ mostfrequentterms.J next mostf
14、requentterms.J next mostfrequentterms.etc.N gaps of 1 each.N/2 gaps of 2 each.N/3 gaps of 3 each.J-row blocksIn the i th of these J-row blocks, we have J rows each with N/i gaps of i each.Encoding a gap of i takes us 2log2 i +1 bits.So such a row uses space (2N log2 i )/i bits. For the entire block,
15、 (2N J log2 i )/i bits, which in our case is 1.5 x 108 (log2 i )/i bits. Sum this over i from 1 up to m/J = 500K/76 6500. (Since there are m/J blocks.)ExerciseWork out the above sum and show it adds up to about 53 x 150 Mbits, which is about 1GByte.So weve taken 6GB of text and produced from it a 1G
16、B index that can handle Boolean queries!Neat!Make sure you understand all the approximations in our probabilistic calculation.CaveatsThis is not the entire space for our index:does not account for dictionary storage next up;as we get further, well store even more stuff in the index.Analysis assumes
17、Zipfs law model applies to occurrence of terms in docs.All gaps for a term are taken to be the same!Does not talk about query processing.More practical caveat: alignmentg codes are neat in theory, but, in reality, machines have word boundaries 8, 16, 32 bitsCompressing and manipulating at individual
18、 bit-granularity is overkill in practiceSlows down query processing architectureIn practice, simpler byte/word-aligned compression is betterSee Scholer et al., Anh and Moffat referencesFor most current hardware, bytes are the minimal unit that can be very efficiently manipulatedSuggests use of varia
19、ble byte codeVariable-byte integersVariableByteInt_t: A variable-length format for positive integers, defined as where the high-order bit of each byte indicates whether the last byte is reached, and the low-order seven bits are appended as increasingly more significant bits in the resulting integer
20、value. Thus values from zero to 127 may be stored in a single byte, values from 128 to 16,383 may be stored in two bytes, and so on. Variable-byte integer examplesValue First byte Second byte Third byte 012. 127128129130. 16,38316,384 16,385. Variable-byte integer examplesValue First byte Second byt
21、e Third byte 0 100000001 100000012 10000010. 127 11111111128 00000000 10000001129 00000001 10000001130 00000010 10000001. 16,383 01111111 1111111116,384 00000000 00000000 1000000116,385 00000001 00000000 10000001. Byte-aligned compressionUsed by many commercial/research systemsGood low-tech blend of
22、 variable-length coding and sensitivity to alignment issuesFix a word-width of, here, w = 8 bits.Dedicate 1 bit (high bit) to be a continuation bit c.If the gap G fits within (w 1) = 7 bits, binary-encode it in the 7 available bits and set c = 0. Else set c = 1, encode low-order (w 1) bits, and then
23、 use one or more additional words to encode G/2w1 using the same algorithmExerciseHow would you adapt the space analysis for g-coded indexes to the variable byte scheme using continuation bits?Exercise (harder)How would you adapt the analysis for the case of positional indexes?Intermediate step: for
24、get compression. Adapt the analysis to estimate the number of positional postings entries.Word-aligned binary codesMore complex schemes indeed, ones that respect 32-bit word alignment are possibleByte alignment is especially inefficient for very small gaps (such as for commonest words)Say we now use
25、 32 bit word with 2 control bitsSketch of an approach:If the next 30 gaps are 1 or 2 encode them in binary within a single wordIf next gap 215, encode just it in a wordFor intermediate gaps, use intermediate strategiesUse 2 control bits to encode coding strategyDictionary and postings filesUsually i
26、n memoryGap-encoded,on diskInverted index storage We have estimated postings storageNext up: Dictionary storageDictionary is in main memory, postings on diskThis is common, and allows building a search engine with high throughputBut for very high throughput, one might use distributed indexing and ke
27、ep everything in memoryAnd in a lower throughput situation, you can store most of the dictionary on disk with a small, inmemory indexTradeoffs between compression and query processing speedCascaded family of techniquesHow big is the lexicon V?Grows (but more slowly) with corpus sizeEmpirically okay
28、model: Heaps Lawm = kTbwhere b 0.5, k 30100; T = # tokensFor instance TREC disks 1 and 2 (2 GB; 750,000 newswire articles): 500,000 termsm is decreased by case-folding, stemmingIndexing all numbers could make it extremely large (so usually dont)Spelling errors contribute a fair bit of sizeExercise:
29、Can onederive this from Zipfs Law?Dictionary storage - first cutArray of fixed-width entries500,000 terms; 28 bytes/term = 14MB.Allows for fast binarysearch into dictionary20 bytes4 bytes eachExercisesIs binary search really a good idea?What are the alternatives?Fixed-width terms are wastefulMost of
30、 the bytes in the Term column are wasted we allot 20 bytes for 1 letter terms.And we still cant handle supercalifragilisticexpialidocious.Written English averages 4.5 characters/word.Exercise: Why is/isnt this the number to use for estimating the dictionary size?Ave. dictionary word in English: 8 ch
31、aractersShort words dominate token counts but not type average.Compressing the term list: Dictionary-as-a-String.systilesyzygeticsyzygialsyzygyszaibelyiteszczecinszomo.Binary searchthese pointersTotal string length =500K x 8B = 4MBPointers resolve 4Mpositions: log24M =22bits = 3bytesStore dictionary
32、 as a (long) string of characters:Pointer to next word shows end of current wordHope to save up to 60% of dictionary space.Total space for compressed list4 bytes per term for Freq.4 bytes per term for pointer to Postings.3 bytes per term pointerAvg. 8 bytes per term in term string500K terms 9.5MB No
33、w avg. 11 bytes/term, not 20.BlockingStore pointers to every kth term string.Example below: k=4.Need to store term lengths (1 extra byte).7systile9syzygetic8syzygial6syzygy11szaibelyite8szczecin9szomo. Save 9 bytes on 3 pointers.Lose 4 bytes onterm lengths.NetWhere we used 3 bytes/pointer without bl
34、ocking3 x 4 = 12 bytes for k=4 pointers,now we use 3+4=7 bytes for 4 pointers.Shaved another 0.5MB; can save more with larger k.Why not go with larger k?ExerciseEstimate the space usage (and savings compared to 9.5MB) with blocking, for block sizes of k = 4, 8 and 16.Impact on searchBinary search do
35、wn to 4-term block;Then linear search through terms in block.8 documents: binary tree ave. = 2.6 comparesBlocks of 4 (binary tree), ave. = 3 compares = (1+22+43+4)/8 =(1+22+23+24+5)/83757432864281651ExerciseEstimate the impact on search performance (and slowdown compared to k=1) with blocking, for b
36、lock sizes of k = 4, 8 and 16.Total spaceBy increasing k, we could cut the pointer space in the dictionary, at the expense of search time; space 9.5MB 8MBNet postings take up most of the spaceGenerally kept on diskDictionary compressed in memoryExtreme compression (see MG)Front-coding:Sorted words commonly have long common prefix store differences only(for last k-1 in a block of k)8automata8automate9automatic10automation8automata1e2ic3ionEncodes automatExtra lengthbeyond automat.Begins to resemble
温馨提示
- 1. 本站所有资源如无特殊说明,都需要本地电脑安装OFFICE2007和PDF阅读器。图纸软件为CAD,CAXA,PROE,UG,SolidWorks等.压缩文件请下载最新的WinRAR软件解压。
- 2. 本站的文档不包含任何第三方提供的附件图纸等,如果需要附件,请联系上传者。文件的所有权益归上传用户所有。
- 3. 本站RAR压缩包中若带图纸,网页内容里面会有图纸预览,若没有图纸预览就没有图纸。
- 4. 未经权益所有人同意不得将文件中的内容挪作商业或盈利用途。
- 5. 人人文库网仅提供信息存储空间,仅对用户上传内容的表现方式做保护处理,对用户上传分享的文档内容本身不做任何修改或编辑,并不能对任何下载内容负责。
- 6. 下载文件中如有侵权或不适当内容,请与我们联系,我们立即纠正。
- 7. 本站不保证下载资源的准确性、安全性和完整性, 同时也不承担用户因使用这些下载资源对自己和他人造成任何形式的伤害或损失。
最新文档
- 幼儿园早阅课题组下学期工作计划
- 学科竞赛辅导班行业跨境出海项目商业计划书
- 汽车维修与保养培训行业跨境出海项目商业计划书
- 博物馆展陈空间设计企业制定与实施新质生产力项目商业计划书
- 电商产品360度拍摄行业跨境出海项目商业计划书
- 农场直供有机餐厅行业深度调研及发展项目商业计划书
- 滑雪装备电商平台企业制定与实施新质生产力项目商业计划书
- 创意广告设计与制作中心行业跨境出海项目商业计划书
- 智能化合成革供应链管理行业深度调研及发展项目商业计划书
- 电子出版物出版AI应用行业深度调研及发展项目商业计划书
- 国家开放大学本科《管理英语3》一平台机考真题及答案总题库珍藏版
- 20万吨高塔造粒颗粒硝酸铵工艺安全操作规程
- CJJ82-2012 园林绿化工程施工及验收规范
- 江苏省南京市2022-2023学年四年级下学期数学期末试卷(含答案)
- 江苏省南京市建邺区2022-2023学年五年级下学期期末数学试卷
- 提高感染性休克集束化治疗完成率工作方案
- 肝硬化病人健康宣教课件
- 心力衰竭病人的护理课件
- 0-3岁儿童适应性行为的发展与教育
- 【多功能自动跑步机机械结构设计4800字(论文)】
- 动物生理学血细胞计数实验报告
评论
0/150
提交评论