

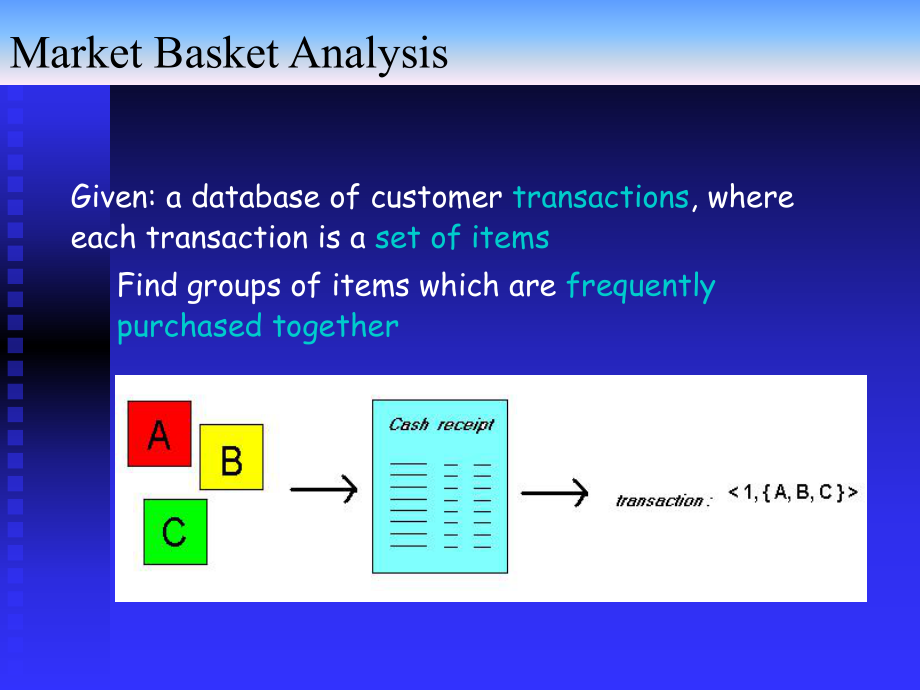


版权说明:本文档由用户提供并上传,收益归属内容提供方,若内容存在侵权,请进行举报或认领
文档简介
1、 Customer buying habits by finding associations and correlations between the different items that customers place in their “shopping basket” Customer1Customer2Customer3Milk, eggs, sugar, breadMilk, eggs, cereal, bread Eggs, sugarMarket Basket Analysis (MBA)Given: a database of customer transactions,
2、 where each transaction is a set of itemsFind groups of items which are frequently purchased together Market Basket AnalysisMBA applicable whenever a customer purchases multiple things in proximity Goal of MBAAssociation RulesTransactions:Relational formatCompact formatItem: single element, Itemset:
3、 set of itemsSupport of an itemset I denoted by sup(I): card(I)Threshold for minimum support: Itemset I is Frequent if: sup(I) .Frequent Itemset represents set of items which arepositively correlatedBasic Concepts itemset sup(dairy) = 3 sup(fruit) = 3 sup(dairy, fruit) = 2 If = 3, then dairy and fru
4、it are frequent while dairy,fruit is not. Customer 1 Customer 2Frequent ItemsetsTransaction IDItems Bought1dairy,fruit2dairy,fruit, vegetable3dairy4fruit, cerealsq A,B - partition of a set of items q r = A B Support of r: sup(r) = sup(AB)Confidence of r: conf(r) = sup(AB)/sup(A)q Thresholds:u minimu
5、m support - su minimum confidence cr AS(s, c), if sup(r) s and conf(r) cAssociation Rules: AR(s,c)Transaction IDItems Bought2000A,B,C1000A,C4000A,D5000B,E,FFrequent Itemset SupportA75%B50%C50%A,C50%Min. support 2 50%Min. confidence - 50%Association Rules - ExampleThe Apriori algorithm Agrawalabcdc,
6、db, db, ca, da, ca, ba, b, db, c, da, c, da, b, ca,b,c,da,d is not frequent, so the 3-itemsets a,b,d, a,c,d and the 4-itemset a,b,c,d, are not generated.Apriori - ExampleAlgorithm Apriori: IllustrationuMining association rules is composed of two steps:TID Items1000 A, B, C2000 A, C3000 A, D4000 B, E
7、, F1. discover the large items, i.e., the sets of itemsets that have transaction support above a predetermined minimum support s.2. Use the large itemsets to generate the association rules A 3 B 2C 2A,C 2 Large support itemsMinSup = 2TID Items100 A, C, D200 B, C, E300 A, B, C, E400 B, E Database DA
8、B C D E Itemset CountA 2 B 3C 3E 3Itemset CountA, B A, C A, E B, C B, EC, E Itemset A,B A,C A,E B,C B,E C,E Itemset Count A, C 2 B, C 2 B, E 3C, E 2 Itemset Count B, C, E Itemset B, C, E 2 Itemset Count B, C, E 2 Itemset Count C1F1C2F2C2C3F3C3ScanDScanDScanDS = 22 3 3 1 3 1 2 1 2 3 2 Representative
9、Association RulesTransactions:A,B,C,D,EA,B,C,D,E,FA,B,C,D,E,H,IA,B,EB,C,D,E,H,IRepresentative Association RulesFind RR(2,80%)Representative Rules From (BCDEHI): H B,C,D,E,I I B,C,D,E,HFrom (ABCDE):A,C B,D,EA,D B,C,ETransactions:abcdeabcacdebcdebcbdecdeFrequent Pattern (FP) Growth StrategyMinimum Sup
10、port = 2Frequent Items:c 6b 5d 5e 5a 3Transactionsordered:cbdeacbacdeacbdecbbdecdeFP-treeFrequent Pattern (FP) Growth StrategyMining the FP-tree for frequent itemsets:Start from each item and construct a subdatabase of transactions (prefix paths) with that item listed at the end. Reorder the prefix
11、paths in support descending order. Build a conditional FP-tree.a 3 Prefix path:(c b d e a, 1)(c b a, 1)(c d e a, 1)Correct order:c 3b 2d 2e 2Frequent Pattern (FP) Growth Strategya 3 Prefix path:(c b d e a, 1)(c b a, 1)(c d e a, 1)Frequent Itemsets:(c a, 3)(c b a, 2)(c d a, 2)(c d e a, 2)(c e a, 2)Mu
12、ltidimensional ARAssociations between values of different attributes :RULES:nationality = French income = high 50%, 100%income = high nationality = French 50%, 75%age = 50 nationality = Italian 33%, 100%Multi-dimensional Single-dimensional Schema: Single-dimensional AR vs Multi-dimensionalQuantitati
13、ve AttributesProblem: too many distinct valuesSolution: transform quantitative attributes into categorical ones via discretization. Discretization of quantitative attributesConstraint-based ARApriori property revisitedMining Association Rules with ConstraintsMultilevel ARProductFam ilySectorDepartm
14、entF ro z e nR e frig e ra te dV e g e ta b leB a n a n a A p p le O ra n g e E tc .F ru itD a iryE tc .F re s hB a k e ryE tc .F o o d S tu ffHierarchy of conceptsFreshsupport = 20%Dairy support = 6%Fruit support = 1%Vegetable support = 7%q Support and Confidence of Multilevel Association RulesHier
15、archical attributes: age, salaryAssociation Rule: (age, young) (salary, 40k) ageyoung middle-aged old salarylow medium high 18 29 30 60 61 8010k40k 50k 60k 70k 80k100kCandidate Association Rules: (age, 18 ) (salary, 40k), (age, young) (salary, low), (age, 18 ) (salary, low)Mining Multilevel ARMining Multilevel ARMulti-level Assoc
温馨提示
- 1. 本站所有资源如无特殊说明,都需要本地电脑安装OFFICE2007和PDF阅读器。图纸软件为CAD,CAXA,PROE,UG,SolidWorks等.压缩文件请下载最新的WinRAR软件解压。
- 2. 本站的文档不包含任何第三方提供的附件图纸等,如果需要附件,请联系上传者。文件的所有权益归上传用户所有。
- 3. 本站RAR压缩包中若带图纸,网页内容里面会有图纸预览,若没有图纸预览就没有图纸。
- 4. 未经权益所有人同意不得将文件中的内容挪作商业或盈利用途。
- 5. 人人文库网仅提供信息存储空间,仅对用户上传内容的表现方式做保护处理,对用户上传分享的文档内容本身不做任何修改或编辑,并不能对任何下载内容负责。
- 6. 下载文件中如有侵权或不适当内容,请与我们联系,我们立即纠正。
- 7. 本站不保证下载资源的准确性、安全性和完整性, 同时也不承担用户因使用这些下载资源对自己和他人造成任何形式的伤害或损失。
最新文档
- 鲁教版地理六年级下册6.1《位置和范围》听课评课记录3
- 【部编人教版】八年级上册历史听课评课记录 第11课 北洋政府的黑暗统治
- 浙教版数学七年级上册5.2《解法》听评课记录
- 环境监测承包协议书
- 人教版地理八年级上册《第一节 交通运输》听课评课记录1
- 沪教版数学八年级下册20.2《一次函数的图象与性质》听评课记录1
- 湘教版数学九年级下册《1.5 二次函数的应用》听评课记录1
- 六年级科学斜面听评课记录
- 新北师大版数学一年级下册《采松果》听评课记录
- 苏科版数学九年级上册听评课记录 用一元二次方程解决问题
- 北京理工大学应用光学课件(大全)李林
- 国家综合性消防救援队伍消防员管理规定
- 河南省三门峡市各县区乡镇行政村村庄村名居民村民委员会明细
- 2023年全国各地高考英语试卷:完形填空汇编(9篇-含解析)
- 五年级上册数学习题课件 简便计算专项整理 苏教版 共21张
- 疼痛科的建立和建设
- 运动技能学习PPT课件
- 第六编元代文学
- 高考语文古诗词必背重点提纲
- 超星尔雅学习通《大学生心理健康教育(兰州大学版)》章节测试含答案
- 2020译林版高中英语选择性必修二单词默写表
评论
0/150
提交评论